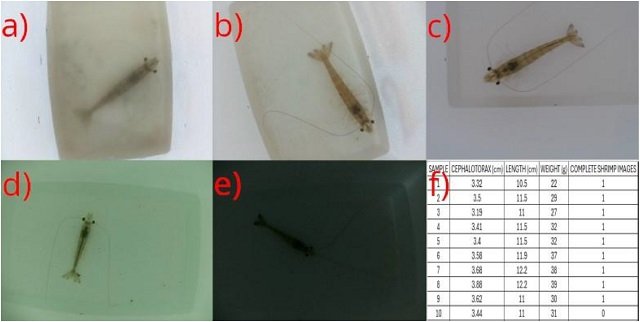
Traditionally, shrimp farming relies on manual processes to estimate pond biomass (the total weight of shrimp in a pond). This method is slow, prone to errors, and ultimately limits farm efficiency. However, a new wave of innovation is on the horizon, driven by advances in computer vision (CV) and artificial intelligence (AI).
A recently published dataset in the journal Data in Brief by scientists from the National Technological Institute of Mexico and the Sonora State University, available for free to researchers, paves the way for the development and testing of sophisticated models that can accurately detect and measure shrimp, leading to a more precise and streamlined approach to biomass estimation.
Precision Aquaculture: A Data-Driven Approach
Accurate shrimp biomass estimation is a cornerstone of precision aquaculture. By understanding the total weight of shrimp in a pond, farmers can optimize feed management, reduce operational costs, and minimize environmental impact. However, traditional biomass estimation methods often involve invasive techniques that can stress and harm shrimp populations.
The Problem: Deficiencies of Manual Measurement
Current methods for estimating shrimp biomass involve physically capturing samples, measuring them individually, and extrapolating the results to estimate the total pond weight. This slow and disruptive process introduces inaccuracies and can negatively affect shrimp health. Additionally, relying solely on manual measurements limits the ability to collect data frequently, making real-time decision-making for farm management challenging.
Inaccuracies in shrimp measurements can create a domino effect of problems, including:
- Inefficient feeding: Overfeeding or underfeeding can negatively impact shrimp health, water quality, and production costs.
- Delayed harvests: Inaccurate biomass estimates can result in delayed harvests, affecting both shrimp quality and market opportunities.
- Unnecessary stress: Repeated physical handling can stress shrimp, which can hinder their growth and affect the overall productivity of the farm.
The limitations of current methods have driven the development of non-invasive approaches. These techniques aim to estimate shrimp biomass without physically capturing and weighing individual animals. Computer vision (CV) and artificial intelligence (AI) offer promising solutions, but they require large and diverse datasets to train effective models.
The Solution: A Dataset for AI Innovation
The new dataset addresses this challenge by providing a comprehensive collection of shrimp images and corresponding manual measurements. Below is a breakdown of the data:
- Image capture: Over 5,500 images of shrimp were captured in controlled environments with constant water levels.
- Shrimp samples: The total length, cephalothorax length, and weight of a total of 170 individual shrimp were measured.
- Data organization: The dataset is conveniently divided into five subfolders, with corresponding Excel files containing detailed manual measurements.
- Diverse farming conditions: Images were captured of shrimp raised in both industrial farms and university research environments, providing valuable insights into variations in shrimp size and appearance.
Unlocking the Potential of AI and CV
This rich dataset provides researchers and developers with a powerful tool to explore a variety of approaches:
- Computer vision models: The image data can be used to train CV models that automatically detect individual shrimp within a pond image.
- AI-driven biomass estimation: Combining shrimp detection through CV with AI algorithms can lead to the development of models that accurately calculate total pond biomass based solely on images.
- Machine learning with manual data: Machine learning models can leverage the detailed measurements in the dataset to explore correlations between shrimp size and weight, potentially leading to more efficient biomass estimation even without image analysis.
Benefits for the Shrimp Farming Industry
The successful development of AI-driven biomass estimation models will revolutionize shrimp farming by:
- Increased accuracy: Precise pond biomass data will lead to more informed management decisions regarding feeding, harvesting, and overall farm optimization.
- Reduced costs: Optimized data collection methods will save time and resources, improving farm efficiency.
- Improved animal welfare: The reduced need for manual sampling will minimize stress on shrimp populations.
- Data-driven decision-making: Real-time biomass data will enable farmers to make data-based decisions to enhance sustainability and profitability.
- Optimized feeding strategies: Accurate biomass estimation allows for customized feeding plans, minimizing waste and maximizing shrimp health.
The dataset offers a valuable resource for researchers and developers working on computer vision and machine learning models for shrimp biomass estimation. It also provides a foundation for comparing the effectiveness of these models with traditional techniques that rely solely on manually extracted features.
The Future of Shrimp Farming
The release of this dataset marks an important milestone in the development of AI-driven biomass estimation for shrimp farming. By providing researchers with a rich and diverse dataset, this resource can accelerate the development of innovative solutions that will transform the aquaculture industry.
The study was partially funded by CONAHCYT and the National Technological Institute of Mexico.
Contact
Edgard Esquer-Miranda
Universidad Estatal de Sonora
Av. Ley Federal del Trabajo S/N, Hermosillo, Sonora C.P. 83100, México
Email: edgard.esquer@ues.mx
Reference (open access)
Ramírez-Coronel, F. J., Esquer-Miranda, E., Rodríguez-Elias, O. M., García-Hinostro, P., & Parra-Salazar, G. C. (2024). A Litopenaeus vannamei shrimp dataset for artificial intelligence-based biomass estimation and organism detection algorithms. Data in Brief, 57, 110964. https://doi.org/10.1016/j.dib.2024.110964