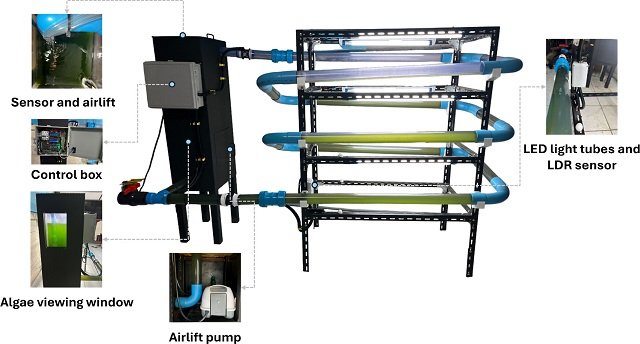
Microalgae have immense potential as a sustainable resource for various applications, including biofuels, food supplements, and pharmaceuticals. However, traditional cultivation methods often face challenges related to scalability, efficiency, and environmental impact. To address these limitations, researchers are increasingly turning to innovative technologies to optimize microalgae production.
Researchers from Khon Kaen University and Kasetsart University have taken a significant step in this direction by developing a cutting-edge, smart cultivation system for the microalga Chlorella sp. The system integrates a closed tubular photobioreactor (PBR) with advanced technologies such as computational fluid dynamics (CFD), the Internet of Things (IoT), artificial intelligence (AI), and image processing.
Challenges in Microalgae Cultivation
Despite their immense potential, microalgae cultivation is not without challenges. Traditional cultivation methods often encounter issues such as contamination, nutrient limitation, and suboptimal growth conditions. In this sense, successful production heavily depends on optimizing growth conditions and meticulous monitoring of key parameters to ensure optimal biomass production.
Advanced cultivation systems, such as photobioreactors (PBRs), have emerged as pioneers in controlled microalgae cultivation technology. Tubular PBRs, in particular, offer significant advantages over traditional open pond systems, including a lower risk of contamination, better control over growth conditions, and substantially higher biomass yields.
The Power of Technology
Recent advancements in technology have opened new possibilities for optimizing microalgae cultivation. Integrating Internet of Things (IoT) devices, artificial intelligence (AI), and image processing techniques has the potential to revolutionize the industry.
- IoT-enabled Monitoring: IoT sensors can continuously monitor critical parameters such as temperature, pH, light intensity, and nutrient levels. By collecting real-time data, growers can make informed decisions to optimize growth conditions.
- AI-driven Optimization: AI algorithms, such as machine learning, can analyze historical data to identify patterns and predict future trends. This enables predictive modeling and automated control of cultivation parameters, leading to better efficiency and productivity.
- Image Processing for Growth Assessment: Image processing techniques can be used to non-invasively monitor microalgae growth by analyzing changes in cell density, morphology, and color. This provides valuable insights into the cultivation process and allows for timely adjustments.
Real-time Monitoring and Management
The key innovation lies in the development of a closed tubular photobioreactor (PBR) designed with computational fluid dynamics (CFD), Internet of Things (IoT), artificial intelligence (AI), and image processing technologies.
A network of sensors continuously monitors critical parameters such as temperature, pH, light intensity, electrical conductivity, flow rate, oxygen content, and light exposure duration. This real-time data is processed by an AI-driven system to optimize growth conditions and prevent potential issues.
This system enables non-invasive, real-time observation and management of algae growth, eliminating the need for manual sampling and reducing contamination risks.
Sensor Integration and Data Analysis
Seven types of sensors were installed in the PBR to monitor key characteristics such as temperature, pH, light intensity, electrical conductivity (EC), flow rate, oxygen content, and light exposure duration. An ESP8266 microcontroller was used as the main control unit to manage sensor data and system operations. Additionally, an ESP32 camera took images at a resolution of 33 × 33 pixels at 30-minute intervals.
By analyzing the color intensity of the images, the system can accurately estimate algae density without the need for destructive sampling.
Machine Learning Models and Predictive Analysis
The collected data was used to train machine learning (ML) models, aiming to forecast and improve agricultural scenarios. The dataset consisted of 602 samples, evenly distributed between 12-hour and 24-hour light cycles. The results showed significant improvements in biomass productivity, with a constant 24-hour light exposure producing a 7.19% increase, compared to a 2.09% increase observed in the 12-hour cycle.
Feature Importance and Model Evaluation
Feature importance analysis revealed that temperature and light intensity are the most significant parameters for growth. The eXtreme Gradient Boosting (XGBoost) model demonstrated remarkable efficacy in growth projection, achieving an R2 value of 0.9997 for the training dataset.
Conclusion
This innovative research demonstrates the power of smart technology to revolutionize microalgae cultivation. By combining advanced sensors, AI, and machine learning, researchers have developed a system that can significantly enhance the efficiency, sustainability, and scalability of microalgae production. This has far-reaching implications for the development of renewable energy sources, sustainable food production, and environmental restoration.
Reference (open access)
Tummawai, T., Rohitatisha Srinophakun, T., Padungthon, S., & Sukpancharoen, S. (2024). Application of Artificial Intelligence and Image Processing for the Cultivation of Chlorella sp. Using Tubular Photobioreactors. ACS Omega.