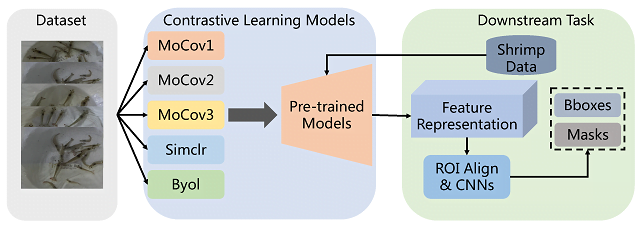
Recent advancements in shrimp farming have focused on sustainability, productivity, and efficiency. However, with the advancement in information technologies, the concept of ‘smart shrimp farming’ has emerged.
The approach of ‘smart shrimp farming’ is an innovative application of advanced technologies and innovative management practices in shrimp aquaculture.
Current technologies assist shrimp farmers in managing the entire process of shrimp aquaculture. These advanced techniques enable real-time monitoring of shrimp growth and water quality, feed management, and disease outbreak detection.
Computer vision techniques can automate various aspects of shrimp farming using cameras and image processing or deep learning methods.
In this regard, researchers from Jeonbuk National University, Korea National University of Agriculture and Fisheries, and Daesang Aquaculture Trout Association Corporation propose an innovative approach to bridge the gap between computer vision technology and shrimp aquaculture through the use of unsupervised learning to train deep learning models.
Specifically, the study suggests using a contrastive learning (CL) mechanism to train the feature extractor and obtain visual representations of shrimp.
Applications of computer vision in shrimp aquaculture
Shrimp counting is a widely applied computer vision technology in the field of shrimp farming. The researchers propose an automatic shrimp counting method using the YOLO algorithm.
On the other hand, other researchers propose YOLOv4 and MobileNetv3 for counting shrimp throughout the entire cultivation process.
Finally, the Deep-ShrimpNet model classifies shrimp and evaluates their quality.
Implementation Details
“Our implementation was based on the open-source tool MMSelfSup. We conducted all the experiments on contrastive learning models using this tool,” they report.
Training contrastive learning models is typically referred to as a pretext task, which is a self-supervised learning task aimed at learning visual representations.
Firstly, the researchers constructed a database containing two sub-databases, where the first database has around 10,000 unlabeled shrimp images that can be used for unsupervised training of the learning models.
The second sub-database contains a thousand shrimp images with high-quality labeled information.
Subsequently, the researchers trained five contrastive learning models, including MoCov1, MoCov2, MoCov3, SimCLR, and Byol, and used the results to pretrain the Mask R-CNN model.
Conclusions
“We propose a novel approach to integrate state-of-the-art unsupervised learning with shrimp farming to reduce dependency on labeled data in shrimp instance segmentation,” they conclude.
Additionally, the researchers summarize the study’s findings as follows:
The study contributes a publicly available dataset of high-quality shrimp images. It also includes a database of shrimp segments to support the development of deep learning methods.
It introduces a new approach that provides a novel way to combine unsupervised learning methods with shrimp instance segmentation.
The study establishes a new benchmark for shrimp instance segmentation in both supervised and unsupervised learning approaches. The code is published at https://github.com/heng94/ShrimpInstanceSegmentation.git (accessed on June 6, 2023).
The study was funded by the Korea Institute of Marine Science & Technology Promotion (KIMST) of the Ministry of Oceans and Fisheries.
Reference (open access)
Zhou, Heng, Sung Hoon Kim, Sang Cheol Kim, Cheol Won Kim, Seung Won Kang, and Hyongsuk Kim. 2023. “Instance Segmentation of Shrimp Based on Contrastive Learning” Applied Sciences 13, no. 12: 6979. https://doi.org/10.3390/app13126979