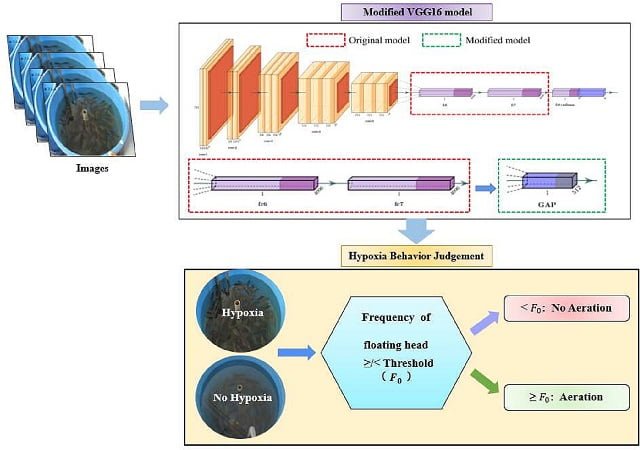
Imagine a fish farm that automatically adjusts oxygen levels based on fish behavior! This futuristic concept is becoming a reality with the help of artificial intelligence (AI) and deep learning.
Recirculating aquaculture systems (RAS) are revolutionizing fish farming, offering a controlled environment for optimal growth. However, current RAS systems cannot learn and self-adjust, leading to inefficiencies in energy use and potentially impacting fish health.
Research conducted by scientists at Chongqing Technology and Business University has designed a precise aeration strategy based on deep learning to improve the healthy growth status of fish. This research adds to the use of artificial intelligence for the economic optimization of RAS.
The challenge: achieving balance
RAS systems rely on aeration to maintain crucial levels of dissolved oxygen (DO) for fish health. However, manual control or fixed thresholds for aeration can lead to a range of wastage. This approach has limitations:
- High costs: Continuous monitoring and adjustments by staff can be costly.
- Inefficiency: Manual controls may not always provide the most optimal aeration levels, resulting in energy waste.
- Suboptimal growth: Inadequate aeration can negatively affect fish growth and overall health.
In summary, excessive aeration consumes unnecessary energy, while insufficient aeration can lead to hypoxia (oxygen deficiency) and hinder fish growth.
The advantage of deep learning: smart aeration
This research presents an innovative solution: a deep learning-based system for precise aeration control. Here’s how it works:
- Computer vision detects hypoxia behavior: Cameras use a modified VGG16 model, a type of deep learning algorithm, to analyze fish movement and identify signs of hypoxia, such as gasping.
- Image segmentation measures fish size: The system segments fish in camera images to calculate the length of their bodies, a key factor in oxygen needs.
- The biological energy model predicts oxygen consumption: The system takes into account fish size, water temperature, and activity levels to estimate how much oxygen fish need.
- AI optimizes aeration strategy: By combining information from the three components, the system generates an adaptive aeration strategy in real-time. This ensures sufficient oxygen for fish without wasting energy on unnecessary aeration.
Real-world results: saving energy, boosting growth
The study compared the proposed deep learning system with traditional methods:
- Energy savings: Compared to manual control and threshold control, the deep learning system achieved a significant reduction in energy consumption: 26.3% and 12.8%, respectively.
- Faster growth: By maintaining optimal DO levels, the system promoted stable water quality and accelerated fish growth. The average production cycle (reaching 400 g in weight) shortened from 5 to 6 months to a notable 3 to 4 months.
A smarter future for aquaculture
This research paves the way for a new era of smart aquaculture. By integrating deep learning with RAS systems, fish farmers can achieve:
- Reduced operating costs: Lower energy consumption translates into significant cost savings.
- Improved fish health: Precise aeration ensures optimal DO levels, minimizing stress and promoting healthy fish growth.
- Shorter production cycles: Faster growth cycles lead to increased productivity and profitability.
Conclusion
This deep learning-based aeration strategy paves the way for a smarter and more sustainable future for RAS aquaculture. By optimizing oxygen levels and minimizing energy waste, this technology benefits both fish farmers (through lower costs and healthier fish) and the environment (through reduced energy consumption).
The study’s innovative approach positions deep learning as a key driver in the future of sustainable and efficient aquaculture. By creating a smarter environment based on real-time water quality monitoring, fish farmers can optimize production while ensuring the welfare of their fish.
The study was funded by the Chongqing Municipal Education Commission, Chongqing Science and Technology Commission, and Chongqing Technology and Business University.
Contact
Yu Shen
National Research Base of Intelligent Manufacturing Services, Chongqing Technology and Business University
Chongqing 400067, China
Email: Shenyu@ctbu.edu.cn
Reference (open access)
Yang, J., Zhou, Y., Guo, Z., Zhou, Y., & Shen, Y. (2024). Deep learning-based intelligent precise aeration strategy for factory recirculating aquaculture systems. Artificial Intelligence in Agriculture. https://doi.org/10.1016/j.aiia.2024.04.001

Editor at the digital magazine AquaHoy. He holds a degree in Aquaculture Biology from the National University of Santa (UNS) and a Master’s degree in Science and Innovation Management from the Polytechnic University of Valencia, with postgraduate diplomas in Business Innovation and Innovation Management. He possesses extensive experience in the aquaculture and fisheries sector, having led the Fisheries Innovation Unit of the National Program for Innovation in Fisheries and Aquaculture (PNIPA). He has served as a senior consultant in technology watch, an innovation project formulator and advisor, and a lecturer at UNS. He is a member of the Peruvian College of Biologists and was recognized by the World Aquaculture Society (WAS) in 2016 for his contribution to aquaculture.