Aquaponics is an innovative approach with the potential to significantly improve crop production while simultaneously promoting sustainability. However, for aquaponic systems to be truly “smart” and efficient, real-time fish monitoring is essential.
In this regard, scientists from the University of Macau, Beijing Jiaotong University, Lingyange Semiconductor Inc., Zhuhai UM Science and Technology Research Institute, and the University of Leeds have designed a new neuromorphic computer called SpikoPoniC. It utilizes spiking neural networks (SNN) to estimate both the length and weight of fish in real-time, addressing the challenges of slow and costly systems with limited scalability.
The Need for Intelligent Aquaponics
Smart aquaponic systems are becoming increasingly crucial in modern aquaculture. The ability to monitor and manage fish growth and well-being in real-time ensures that the system functions optimally, resulting in higher crop yields and overall sustainability.
However, most existing smart aquaponic systems suffer from significant limitations in terms of speed and cost, making large-scale implementation challenging.
“Smart aquaponic systems (SAS) first receive information from sensors that collect data about the environment and then use that information to make critical decisions, such as controlling the level of oxygen and nutrients in the ecosystem,” report the researchers.
Introducing SpikoPoniC: A Turning Point
To address these limitations, researchers have developed an innovative solution: SpikoPoniC. This groundbreaking neuromorphic computer employs spiking neural networks (SNN) to estimate fish size, both in terms of length and weight, in real-time. What sets SpikoPoniC apart from traditional systems is its remarkable speed and cost-effectiveness.
The study presents a novel hardware-efficient SNN-based neuromorphic engine for fish size estimation, applicable in aquaponic monitoring. The system has been modeled in the Verilog language at the register transfer level (RTL).
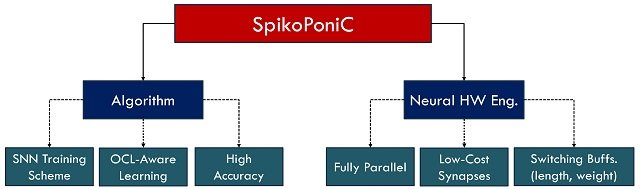
A New Hybrid Training Approach
The core of SpikoPoniC lies in its unique hybrid training scheme. To effectively train the SNN, the system employs a combination of direct SNN backpropagation and standard backpropagation. By doing so, it strikes a balance between high hardware efficiency and accuracy. This blend ensures that SpikoPoniC can provide precise real-time fish monitoring without compromising performance.
Ultra-Fast Classification
“Estimating fish length and weight is important for managing aquaponic systems properly and modeling population trends. The dynamics of fish length distribution are a key input for understanding fish population dynamics and making informed management decisions for exploited populations,” describe the researchers.
The capabilities of SpikoPoniC are truly impressive. It can classify more than 84 million fish samples in just one second, achieving a remarkable speedup of at least 3369 times compared to traditional general-purpose computers. This level of speed not only allows for real-time monitoring but also paves the way for large-scale implementation in aquaponic systems.
Cost-Efficiency and Accessibility
Unlike many SNN-based hardware systems, SpikoPoniC is a cost-effective solution. It consumes less than 1100 slice registers on Virtex 6, making it a highly efficient choice for aquaponics enthusiasts, researchers, and commercial aquaponic facilities. This accessibility makes it a turning point for aquaponics, democratizing the benefits of real-time intelligent monitoring.
Conclusion
Aquaponics represents a promising future for agriculture, combining fish farming and plant cultivation in a mutually beneficial relationship. However, for aquaponics to be truly efficient and scalable, the need for real-time fish monitoring is fundamental.
“This article presents a novel training methodology for training and implementing a spiking neural network in a neuromorphic system for intelligent aquaponics. The article also presents a novel surrogate gradient for SNN training, promising flexibility and hardware efficiency,” conclude the researchers.
SpikoPoniC, a neural network-driven neuromorphic computer, emerges as an innovative solution. Its hybrid training scheme, ultra-fast classification capabilities, cost-effectiveness, and accessibility make it the first of its kind in real-time intelligent aquaponic monitoring.
The study has been funded by Zhuhai UM Science and Technology Research Institute, Lingyange Semiconductor Incorporated, and Blue Ocean Smart System (Nanjing) Limited.
Reference (open access)
Siddique, A.; Sun, J.; Hou, K.J.; Vai, M.I.; Pun, S.H.; Iqbal, M.A. SpikoPoniC: A Low-Cost Spiking Neuromorphic Computer for Smart Aquaponics. Agriculture 2023, 13, 2057. https://doi.org/10.3390/agriculture13112057