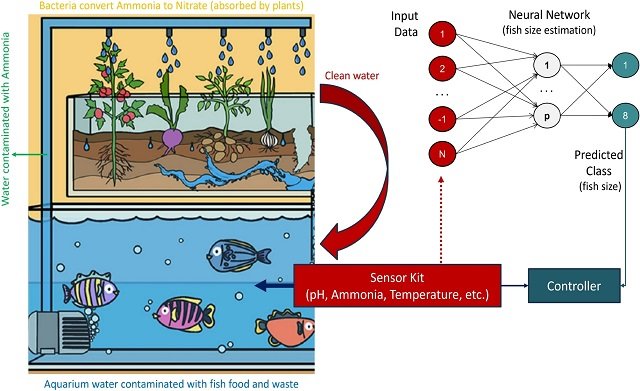
Aquaponics offers a sustainable and efficient solution for producing fresh food. However, successful aquaponics requires maintaining a delicate balance between fish and plants. A key factor that beginners often overlook is the appropriate fish size.
A new study published in Agricultural Research by scientists from the University of Macau, the University of Leeds, Beijing Jiaotong University, and the University of Agriculture explores a novel approach that uses deep learning to estimate fish size in real-time, empowering even novice aquaponics enthusiasts.
The Need for Smart Aquaponics
Traditional aquaponics systems heavily rely on human intervention, which often leads to suboptimal performance. Smart systems, equipped with sensors, data analysis, and automation, can significantly improve efficiency and sustainability. Deep learning, a subset of artificial intelligence, offers powerful tools for predicting various parameters in aquaponics systems, including fish size and water quality.
Challenges and Limitations of Existing Systems
- Software-Based Limitations: Most current smart aquaponics systems depend on traditional software and computing architectures, which can be slow and resource-intensive, especially for large-scale operations.
- Data Scarcity: The lack of datasets makes it difficult to develop and evaluate accurate deep learning models.
- Inexperienced Workforce: The complexity of managing aquaponics systems often requires specialized knowledge and skills.
The Challenge of Inexperienced Management
Aquaponics offers numerous advantages, including chemical-free farming and efficient water use. However, its commercial adoption may be hindered by the need for trained operators to monitor and manage the system. Accurately estimating fish size is crucial to maintaining a stable environment where both fish and plants thrive.
Deep Learning to the Rescue
This article explores a novel approach to addressing the challenge of estimating fish size in aquaponics systems in real-time. Using a technique called deep learning, the researchers have developed a system that can accurately estimate fish size without requiring extensive experience.
The Power of Deep Neural Networks
The system uses a “deep neural network,” a type of artificial intelligence inspired by the human brain. This network is trained on a massive set of images containing fish of various sizes. By analyzing these images, the network learns to identify patterns and correlations between fish size and specific visual features.
Balancing Efficiency with Accuracy
A key aspect of this innovation is the use of a specific function called the “swish function.” While traditional implementations of swish can be computationally expensive, the researchers present a novel, hardware-efficient version here. This advancement enables highly accurate fish size estimation without compromising system performance.
Real-Time Monitoring: Speed and Efficiency
One of the most exciting aspects of this development is the creation of a deep learning accelerator. This specialized hardware can process an astounding 40 million fish samples per second. This translates into a real-time monitoring system that is approximately 1,600 times faster than traditional methods relying on general-purpose computers.
Benefits for Aquaponics Growers
This innovative deep learning approach holds immense potential for the future of aquaponics. By automating fish size estimation, it allows beginners to operate aquaponics systems with greater confidence and efficiency. Additionally, the proposed hardware’s low energy requirements make it a viable option for remote and small-scale aquaponics operations.
The main benefits can be grouped as follows:
- Simplified System Management: Real-time fish size estimation enables anyone to operate an aquaponics system confidently, regardless of prior experience.
- Improved System Stability: Accurate fish size data allows for real-time adjustments, promoting optimal growth for both fish and plants.
- Enhanced Scalability: This technology paves the way for larger, commercially viable aquaponics installations.
The Road Ahead
The research presented here paves the way for smarter and more accessible aquaponics systems. As this technology continues to develop, we can expect even more advancements in real-time monitoring and automated system management. This, in turn, will contribute to the broader adoption of aquaponics as a sustainable and productive method for food production.
Contact
Muhammad Azhar Iqbal
Faculty of Engineering and Physical Sciences, University of Leeds
Leeds, LS2-9JT, United Kingdom
Email: m.a.iqbal1@leeds.ac.uk
Reference (open access)
Siddique, A., Iqbal, M.A., Sun, J. et al. N-AquaRAM: A Cost-Efficient Deep Learning Accelerator for Real-Time Aquaponic Monitoring. Agric Res (2024). https://doi.org/10.1007/s40003-024-00788-6