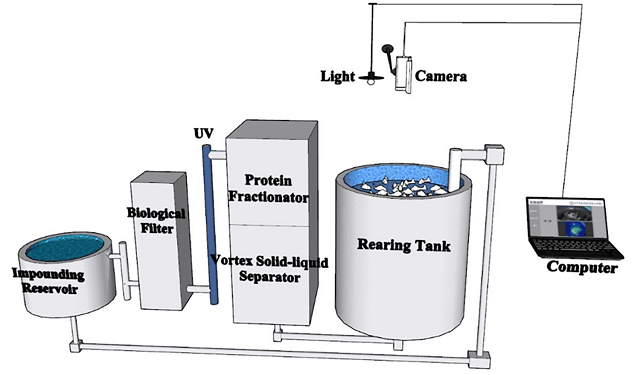
Feeding costs represent a significant portion of expenses in the aquaculture industry. Inefficient feeding practices, often relying on the farmer’s experience, can lead to feed waste and environmental contamination. To optimize feeding strategies, it is essential to accurately assess fish feeding intensity.
To address this issue, computer vision technology offers a promising solution. By analyzing visual data, we can objectively evaluate fish feeding behavior and optimize feed distribution.
An article published by scientists from Dalian Ocean University presents a new computer vision-based approach that utilizes a convolutional neural network (CNN) to accurately assess the feeding intensity of farmed fish.
The Importance of Precise Feeding
Feeding plays a crucial role in aquaculture as it directly impacts fish growth, health, and overall production system efficiency. Overfeeding can lead to feed waste, increased water contamination, and higher production costs. Underfeeding, on the other hand, can hinder growth rates and compromise fish welfare.
Computer Vision: A Promising Solution
Computer vision technology has emerged as a powerful tool to address these challenges in aquaculture. By analyzing visual data, computer vision algorithms can accurately assess fish feeding behavior, enabling more precise feed management. This technology offers several advantages, such as non-invasive monitoring, real-time analysis, and automation potential.
Deep Learning for Feeding Behavior Recognition
Deep learning, a subset of artificial intelligence, has revolutionized computer vision tasks. Convolutional neural networks (CNNs) are particularly well-suited for analyzing visual data, including images and videos of fish. CNNs can automatically learn complex features from data, enabling them to accurately recognize different feeding behaviors.
Proposed Approach
Previous computer vision-based approaches for assessing feeding intensity have been limited in their accuracy and applicability. These methods often rely on a single spatial feature or manual threshold settings, which restrict their effectiveness to specific scenarios. Moreover, the lack of full feature integration hampers their ability to capture the complex dynamics of fish feeding behavior.
To overcome these limitations, the researchers propose an integrated approach that combines computer vision techniques with a CNN. The method incorporates three types of features: temporal, spatial, and statistical data features.
- Temporal features: Optical flow analysis is employed to capture the movement patterns of fish during feeding, providing insights into the intensity and duration of feeding activity.
- Spatial features: Binarization is used to extract the spatial distribution of fish within the feeding area, helping identify feeding hotspots and assess the level of competition for feed.
- Statistical data features: Gray level co-occurrence matrix is used to analyze texture and patterns in feeding images, offering additional insights into the feeding environment and fish behavior.
These features are input into their respective specific feature discrimination networks, and the classification results are fused to build a three-stream network for feeding intensity discrimination.
Results and Discussion
The proposed three-stream network achieved an impressive accuracy of 99.3% in distinguishing feeding intensity, accurately categorizing feeding states as none, weak, or strong. The benefits can be summarized as follows:
- Accuracy: The proposed model achieves an impressive 99.3% accuracy in distinguishing fish feeding states.
- Efficiency: By automating feeding decisions, the model can reduce labor costs and improve operational efficiency.
- Sustainability: Precise feeding practices can help minimize feed waste and reduce water pollution, contributing to a more sustainable aquaculture industry.
- Data-driven decision-making: The model provides valuable insights into fish feeding behavior, enabling data-driven decision-making and optimizing aquaculture operations.
This advancement provides a scientific foundation for intelligent fish feeding in aquaculture and promises to promote the sustainable development of the industry by minimizing feed waste and optimizing environmental impact.
Conclusion
The development of a computer vision-based approach for assessing feeding intensity represents a significant advancement in the aquaculture industry. By accurately evaluating fish feeding behavior, we can optimize feed allocation, reduce feed waste, and minimize environmental impact. This technology has the potential to revolutionize aquaculture practices and promote the sustainable development of the industry.
Contact
Kewei Cai
Key Laboratory of Environment Controlled Aquaculture (Dalian Ocean University) Ministry of Education, Dalian, Liaoning Province, China
College of Mechanical and Electronic Engineering, Dalian Minzu University, Dalian, Liaoning Province, China
Email: caikw0602@live.cn
Reference (open access)
Dong Y, Zhao S, Wang Y, Cai K, Pang H, Liu Y (2024) An integrated three-stream network model for discriminating fish feeding intensity using multi-feature analysis and deep learning. PLoS ONE 19(10): e0310356. https://doi.org/10.1371/journal.pone.0310356

Editor at the digital magazine AquaHoy. He holds a degree in Aquaculture Biology from the National University of Santa (UNS) and a Master’s degree in Science and Innovation Management from the Polytechnic University of Valencia, with postgraduate diplomas in Business Innovation and Innovation Management. He possesses extensive experience in the aquaculture and fisheries sector, having led the Fisheries Innovation Unit of the National Program for Innovation in Fisheries and Aquaculture (PNIPA). He has served as a senior consultant in technology watch, an innovation project formulator and advisor, and a lecturer at UNS. He is a member of the Peruvian College of Biologists and was recognized by the World Aquaculture Society (WAS) in 2016 for his contribution to aquaculture.